RANSAC (
RANdom SAmple Consensus) is an iterative method to estimate parameters
of a certain mathematical model from a set of data which may contain a large number
of outliers. In the context of this algorithm, the noisy points are called
outliers
while non-noisy points (valid points) are called
inliers. Moreover,
RANSAC is a non-deterministic algorithm because it produces a reasonable result
only with a certain probability.
In a different way that other similar algorithms,
RANSAC tries to estimate the
parameters of a certain mathematical model starting from a minimal set of points and
then adding iteratively points contained in a range determined by a threshold value
(
inliers) and discarding the points out of this range (
outliers). The algorithm finishes
when a number of iterations have been executed.
The following point clouds were used in the article
"A Comparative Study of Parallel RANSAC
Implementations in the 3D Space". This article makes a comparative study among different parallel implementations
of the
RANSAC algorithm. All the parallel implementations were written by using OpenMP, POSIX Threads, and CUDA languages.
All of the point clouds are in the
Point Cloud Library
file type (
*.pcd) with format
XYZRGBA and they were captured by using a
Kinect Sensor.
LivingRoom (4.69MB)
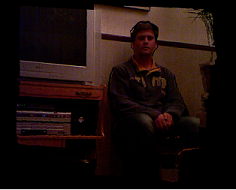
|
Kitchen (4.69MB)
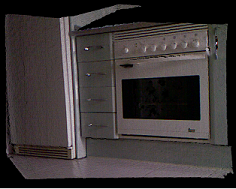
|
Hallway (4.69MB)
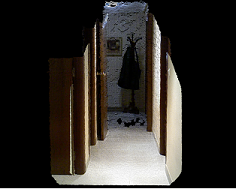
|
|
Furniture (4.69MB)
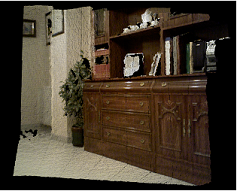
|
Saloon (4.69MB)
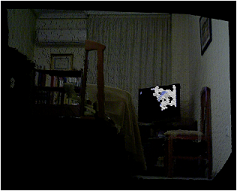
|
Room (4.69MB)
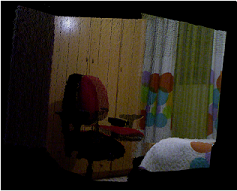
|
Click on the image to download the associate point cloud.
Download all the point clouds.